Análisis de sentimientos en Twitter
Un estudio comparativo
DOI:
https://doi.org/10.51252/rcsi.v3i1.418Palabras clave:
análisis de sentimiento, aprendizaje, clasificación, twitterResumen
El análisis de sentimientos ayuda a determinar la percepción de usuarios en diferentes aspectos de la vida cotidiana, como preferencias de productos en el mercado, nivel de confianza de los usuarios en ambientes de trabajo, o preferencias políticas. La idea es predecir tendencias o preferencias basados en sentimientos. En este artículo evaluamos las técnicas más comunes usadas para este tipo de análisis, considerando técnicas de aprendizaje de máquina y aprendizaje de máquina profundo. Nuestra contribución principal se basa en una propuesta de una estrategia metodológica que abarca las fases de preprocesamiento de datos, construcción de modelos predictivos y su evaluación. De los resultados, el mejor modelo clásico fue SVM, con 78% de precisión, y 79% de métrica F1 (F1 score). Para los modelos de Deep Learning, con mejores resultados fueron los modelos clásicos. El modelo con mejor desempeño fue el de Deep Learning Long Short Term Memory (LSTM), alcanzando un 88% de precisión y 89% de métrica F1. El peor de los modelos de Deep Learning fue el CNN, con 77% de precisión como de métrica F1. Concluyendo que, el algoritmo Long Short Term Memory (LSTM) demostró ser el mejor rendimiento, alcanzando hasta un 89% de precisión.
Citas
Abirami, A. M., & Gayathri, V. (2017). A survey on sentiment analysis methods and approach. 2016 Eighth International Conference on Advanced Computing (ICoAC), 72–76. https://doi.org/10.1109/ICoAC.2017.7951748
Al-Ayyoub, M., Khamaiseh, A. A., Jararweh, Y., & Al-Kabi, M. N. (2019). A comprehensive survey of arabic sentiment analysis. Information Processing & Management, 56(2), 320–342. https://doi.org/10.1016/j.ipm.2018.07.006
Angiani, G., Ferrari, L., Fontanini, T., Fornacciari, P., Iotti, E., Magliani, F., & Manicardi, S. (2016). A comparison between preprocessing techniques for sentiment analysis in Twitter. CEUR Workshop Proceedings, 1748, 1–11. https://ceur-ws.org/Vol-1748/paper-06.pdf
Baby, C. J., Khan, F. A., & Swathi, J. N. (2017). Home automation using IoT and a chatbot using natural language processing. 2017 Innovations in Power and Advanced Computing Technologies (i-PACT), 1–6. https://doi.org/10.1109/IPACT.2017.8245185
Breck, E., & Cardie, C. (2017). Opinion Mining and Sentiment Analysis. In The Oxford Handbook of Computational Linguistics 2nd edition. Oxford University Press. https://doi.org/10.1093/oxfordhb/9780199573691.013.43
Cambria, E. (2016). Affective Computing and Sentiment Analysis. IEEE Intelligent Systems, 31(2), 102–107. https://doi.org/10.1109/MIS.2016.31
Chaturvedi, I., Cambria, E., Welsch, R. E., & Herrera, F. (2018). Distinguishing between facts and opinions for sentiment analysis: Survey and challenges. Information Fusion, 44, 65–77. https://doi.org/10.1016/j.inffus.2017.12.006
Chu, E., & Roy, D. (2017). Audio-Visual Sentiment Analysis for Learning Emotional Arcs in Movies. 2017 IEEE International Conference on Data Mining (ICDM), 829–834. https://doi.org/10.1109/ICDM.2017.100
De Albornoz, J. C., Plaza, L., Gervás, P., & Díaz, A. (2011). A Joint Model of Feature Mining and Sentiment Analysis for Product Review Rating. In Advances in Information Retrieval (pp. 55–66). https://doi.org/10.1007/978-3-642-20161-5_8
Desai, M., & Mehta, M. A. (2016). Techniques for sentiment analysis of Twitter data: A comprehensive survey. 2016 International Conference on Computing, Communication and Automation (ICCCA), 149–154. https://doi.org/10.1109/CCAA.2016.7813707
Dobbin, K. K., & Simon, R. M. (2011). Optimally splitting cases for training and testing high dimensional classifiers. BMC Medical Genomics, 4(1), 31. https://doi.org/10.1186/1755-8794-4-31
Giachanou, A., & Crestani, F. (2016). Like It or Not: A Survey of Twitter Sentiment Analysis Methods. ACM Computing Surveys, 49(2), 1–41. https://doi.org/10.1145/2938640
Go, A., Bhayani, R., & Huang, L. (2009). Twitter Sentiment Classification using Distant Supervision. Processing, 1–6. https://www-cs-faculty.stanford.edu/people/alecmgo/papers/TwitterDistantSupervision09.pdf
Grus, J. (2015). Data Sciemce from Scratch: first principles with python (1st ed.). O’Reilly Media.
Hu, M., & Liu, B. (2004). Mining and summarizing customer reviews. Proceedings of the 2004 ACM SIGKDD International Conference on Knowledge Discovery and Data Mining - KDD ’04, 168. https://doi.org/10.1145/1014052.1014073
Hussein, D. M. E.-D. M. (2018). A survey on sentiment analysis challenges. Journal of King Saud University - Engineering Sciences, 30(4), 330–338. https://doi.org/10.1016/j.jksues.2016.04.002
Jianqiang, Z., & Xiaolin, G. (2017). Comparison Research on Text Pre-processing Methods on Twitter Sentiment Analysis. IEEE Access, 5, 2870–2879. https://doi.org/10.1109/ACCESS.2017.2672677
Kao, A., & Poteet, S. R. (2007). Natural Language Processing and Text Mining (1st ed.). Springer London. https://doi.org/10.1007/978-1-84628-754-1
Kaur, H., Mangat, V., & Nidhi. (2017). A survey of sentiment analysis techniques. 2017 International Conference on I-SMAC (IoT in Social, Mobile, Analytics and Cloud) (I-SMAC), 921–925. https://doi.org/10.1109/I-SMAC.2017.8058315
Kharde, V. A., & Sonawane, S. S. (2016). Sentiment Analysis of Twitter Data: A Survey of Techniques. International Journal of Computer Applications, 139(11), 5–15. https://doi.org/10.5120/ijca2016908625
Li, W., Shao, W., Ji, S., & Cambria, E. (2022). BiERU: Bidirectional emotional recurrent unit for conversational sentiment analysis. Neurocomputing, 467, 73–82. https://doi.org/10.1016/j.neucom.2021.09.057
Liang, B., Su, H., Gui, L., Cambria, E., & Xu, R. (2022). Aspect-based sentiment analysis via affective knowledge enhanced graph convolutional networks. Knowledge-Based Systems, 235, 107643. https://doi.org/10.1016/j.knosys.2021.107643
Lin, P., Luo, X., & Fan, Y. (2020). A Survey of Sentiment Analysis Based on Deep Learning. International Journal of Computer and Information Engineering, 14(12), 473–485. https://publications.waset.org/10011630/a-survey-of-sentiment-analysis-based-on-deep-learning
Liu, B. (2010). Sentiment analysis and subjectivity. Handbook of Natural Language Processing, Second Edition, 2, 627–666. https://www.cs.uic.edu/~liub/FBS/NLP-handbook-sentiment-analysis.pdf
Lovera, F. A., Cardinale, Y. C., & Homsi, M. N. (2021). Sentiment Analysis in Twitter Based on Knowledge Graph and Deep Learning Classification. Electronics, 10(22), 2739. https://doi.org/10.3390/electronics10222739
Lunt, M. (2015). Introduction to statistical modelling: linear regression. Rheumatology, 54(7), 1137–1140. https://doi.org/10.1093/rheumatology/ket146
Martínez Cámara, E., Rodríguez Barroso, N., Moya, A. R., Fernández, J. A., Romero, E., & Herrera, F. (2019). Deep Learning Hyper-parameter Tuning for Sentiment Analysis in Twitter based on Evolutionary Algorithms. 255–264. https://doi.org/10.15439/2019F183
Mikolov, T., Sutskever, I., Chen, K., Corrado, G., & Dean, J. (2013). Distributed representations ofwords and phrases and their compositionality. Advances in Neural Information Processing Systems, 1–10. https://proceedings.neurips.cc/paper/2013/file/9aa42b31882ec039965f3c4923ce901b-Paper.pdf
Mirtalaie, M. A., Hussain, O. K., Chang, E., & Hussain, F. K. (2018). Sentiment Analysis of Specific Product’s Features Using Product Tree for Application in New Product Development. In Advances in Intelligent Networking and Collaborative Systems (8th ed., pp. 82–95). Springer. https://doi.org/10.1007/978-3-319-65636-6_8
Mostafa, M. M. (2013). More than words: Social networks’ text mining for consumer brand sentiments. Expert Systems with Applications, 40(10), 4241–4251. https://doi.org/10.1016/j.eswa.2013.01.019
Oliveira, D. J. S., Bermejo, P. H. de S., & dos Santos, P. A. (2017). Can social media reveal the preferences of voters? A comparison between sentiment analysis and traditional opinion polls. Journal of Information Technology & Politics, 14(1), 34–45. https://doi.org/10.1080/19331681.2016.1214094
Plisson, J., Lavrac, N., & Mladenić, D. D. (2004). A rule based approach to word lemmatization. Proceedings of the 7th International Multiconference Information Society (IS’04), 83–86. http://eprints.pascal-network.org/archive/00000715/
Ribeiro, F. N., Araújo, M., Gonçalves, P., André Gonçalves, M., & Benevenuto, F. (2016). SentiBench - a benchmark comparison of state-of-the-practice sentiment analysis methods. EPJ Data Science, 5(1), 23. https://doi.org/10.1140/epjds/s13688-016-0085-1
Tabinda Kokab, S., Asghar, S., & Naz, S. (2022). Transformer-based deep learning models for the sentiment analysis of social media data. Array, 14, 100157. https://doi.org/10.1016/j.array.2022.100157
Wu, Y., Zhang, Q., Huang, X., & Wu, L. (2011). Structural opinion mining for graph-based sentiment representation. Empirical Methods in Natural Language Processing, 1332–1341. https://aclanthology.org/D11-1123.pdf
Xu, S. (2018). Bayesian Naïve Bayes classifiers to text classification. Journal of Information Science, 44(1), 48–59. https://doi.org/10.1177/0165551516677946
Yujian, L., & Bo, L. (2007). A Normalized Levenshtein Distance Metric. IEEE Transactions on Pattern Analysis and Machine Intelligence, 29(6), 1091–1095. https://doi.org/10.1109/TPAMI.2007.1078
Zhang, L., Wang, S., & Liu, B. (2018). Deep learning for sentiment analysis: A survey. WIREs Data Mining and Knowledge Discovery, 8(4). https://doi.org/10.1002/widm.1253
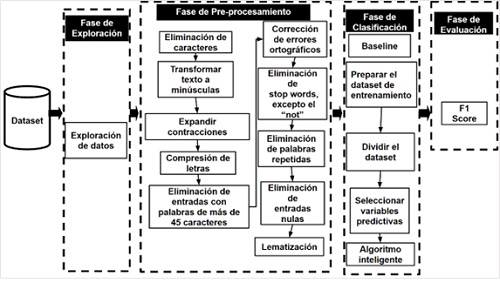
Publicado
Cómo citar
Número
Sección
Licencia
Derechos de autor 2023 Fernando Andres Lovera, Yudith Cardinale

Esta obra está bajo una licencia internacional Creative Commons Atribución 4.0.
Los autores retienen sus derechos:
a. Los autores retienen sus derechos de marca y patente, y tambien sobre cualquier proceso o procedimiento descrito en el artículo.
b. Los autores retienen el derecho de compartir, copiar, distribuir, ejecutar y comunicar públicamente el articulo publicado en la Revista Científica de Sistemas e Informática (RCSI) (por ejemplo, colocarlo en un repositorio institucional o publicarlo en un libro), con un reconocimiento de su publicación inicial en la RCSI.
c. Los autores retienen el derecho a hacer una posterior publicación de su trabajo, de utilizar el artículo o cualquier parte de aquel (por ejemplo: una compilación de sus trabajos, notas para conferencias, tesis, o para un libro), siempre que indiquen la fuente de publicación (autores del trabajo, revista, volumen, número y fecha).