Avances en el aprovechamiento de biopolímeros y productos peruanos
Análisis bibliométrico
DOI:
https://doi.org/10.51252/rcsi.v3i1.489Palabras clave:
redes neuronales, sistemas de recomendación, aprendizaje profundo, nutriciónResumen
El estudio presenta un análisis bibliométrico sobre la aplicación de técnicas de inteligencia artificial en investigaciones relacionadas con hábitos alimentarios. Se analizaron 233 documentos extraídos de la base de datos Scopus desde 1990 hasta 2020, identificando las principales tendencias en la producción científica, fuentes de publicación, afiliaciones institucionales y redes de colaboración. Los resultados muestran un crecimiento exponencial en el número de publicaciones a partir de 2015, atribuible a los avances en IA y al creciente interés en la salud pública. La revista "Lecture Notes in Computer Science" es la fuente con más publicaciones en este campo, seguida por "ACM International Conference Proceeding Series". Las instituciones con mayor producción son el "Weizmann Institute of Science" y la "University of Bari". Asimismo, el análisis de palabras clave destaca la relevancia de técnicas como "machine learning", "deep learning" y "neural networks". Los mapas de colaboración reflejan que Estados Unidos y China son líderes en producción y coautoría. El estudio concluye que la IA ha tenido un impacto creciente en la investigación de hábitos alimentarios, resaltando su importancia como una herramienta emergente para mejorar la comprensión de los hábitos alimentarios y promover intervenciones personalizadas y eficaces en salud pública.
Citas
Aria, M., & Cuccurullo, C. (2017). bibliometrix : An R-tool for comprehensive science mapping analysis. Journal of Informetrics, 11(4), 959-975. https://doi.org/10.1016/j.joi.2017.08.007 DOI: https://doi.org/10.1016/j.joi.2017.08.007
Chen, M., Jia, X., Gorbonos, E., Hong, C. T., Yu, X., & Liu, Y. (2020). Eating healthier: Exploring nutrition information for healthier recipe recommendation. Information Processing and Management, 57(6). https://doi.org/10.1016/j.ipm.2019.05.012 DOI: https://doi.org/10.1016/j.ipm.2019.05.012
Gutiérrez-Esparza, G. O., Ramírez-Delreal, T. A., Martínez-García, M., Infante Vázquez, O., Vallejo, M., & Hernández-Torruco, J. (2021). Machine and deep learning applied to predict metabolic syndrome without a blood screening. Applied Sciences (Switzerland), 11(10). https://doi.org/10.3390/app11104334 DOI: https://doi.org/10.3390/app11104334
Marshall, J., Jimenez-Pazmino, P., Metoyer, R., & Chawla, N. V. (2022). A Survey on Healthy Food Decision Influences Through Technological Innovations. ACM Transactions on Computing for Healthcare, 3(2). https://doi.org/10.1145/3494580 DOI: https://doi.org/10.1145/3494580
McBurney, M. K., & Novak, P. L. (2002). What is bibliometrics and why should you care? Proceedings. IEEE International Professional Communication Conference, 108-114. https://doi.org/10.1109/IPCC.2002.1049094 DOI: https://doi.org/10.1109/IPCC.2002.1049094
Oh, W., An, Y., Min, S., & Park, C. (2022). Comparative Effectiveness of Artificial Intelligence-Based Interactive Home Exercise Applications in Adolescents with Obesity. Sensors, 22(19). https://doi.org/10.3390/s22197352 DOI: https://doi.org/10.3390/s22197352
Pecune, F., Callebert, L., & Marsella, S. (2020). A Socially-Aware Conversational Recommender System for Personalized Recipe Recommendations. Proceedings of the 8th International Conference on Human-Agent Interaction, 78-86. https://doi.org/10.1145/3406499.3415079 DOI: https://doi.org/10.1145/3406499.3415079
Pecune, F., Callebert, L., & Marsella, S. (2022). Designing Persuasive Food Conversational Recommender Systems With Nudging and Socially-Aware Conversational Strategies. Frontiers in Robotics and AI, 8. https://doi.org/10.3389/frobt.2021.733835 DOI: https://doi.org/10.3389/frobt.2021.733835
Ramos, R. G., Domingo, J. D., Zalama, E., Gómez-García-Bermejo, J., & López, J. (2022). SDHAR-HOME: A Sensor Dataset for Human Activity Recognition at Home. Sensors, 22(21). https://doi.org/10.3390/s22218109 DOI: https://doi.org/10.3390/s22218109
Sami, O., Elsheikh, Y., & Almasalha, F. (2021). The Role of Data Pre-processing Techniques in Improving Machine Learning Accuracy for Predicting Coronary Heart Disease. International Journal of Advanced Computer Science and Applications, 12(6), 816-824. https://doi.org/10.14569/IJACSA.2021.0120695 DOI: https://doi.org/10.14569/IJACSA.2021.0120695
Shams, M. Y., Elzeki, O. M., Abouelmagd, L. M., Hassanien, A. E., Elfattah, M. A., & Salem, H. (2021). HANA: A Healthy Artificial Nutrition Analysis model during COVID-19 pandemic. Computers in Biology and Medicine, 135. https://doi.org/10.1016/j.compbiomed.2021.104606 DOI: https://doi.org/10.1016/j.compbiomed.2021.104606
Sujatha, R., Chatterjee, J. M., Thorunitha, S. S., & Nadhiya, S. (2022). Evaluation of Dietary Habits in Relation to Covid-19 Mortality Rate Using Machine Learning Techniques. Journal of System and Management Sciences, 12(2), 174-194. https://doi.org/10.33168/JSMS.2022.0208 DOI: https://doi.org/10.33168/JSMS.2022.0208
Swain, D., Parmar, B., Shah, H., Gandhi, A., Pradhan, M. R., Kaur, H., & Acharya, B. (2022). Cardiovascular Disease Prediction using Various Machine Learning Algorithms. Journal of Computer Science, 18(10), 993-1004. https://doi.org/10.3844/jcssp.2022.993.1004 DOI: https://doi.org/10.3844/jcssp.2022.993.1004
Tian, Y., Zhang, C., Metoyer, R., & Chawla, N. V. (2022). Recipe Recommendation With Hierarchical Graph Attention Network. Frontiers in Big Data, 4. https://doi.org/10.3389/fdata.2021.778417 DOI: https://doi.org/10.3389/fdata.2021.778417
Xie, Y., Jiang, R., Guo, X., Wang, Y., Cheng, J., & Chen, Y. (2022). mmEat: Millimeter wave-enabled environment-invariant eating behavior monitoring. Smart Health, 23. https://doi.org/10.1016/j.smhl.2021.100236 DOI: https://doi.org/10.1016/j.smhl.2021.100236
Zhou, J. (2022). Design of Residents’ Sports Nutrition Data Monitoring System Based on Genetic Algorithm. Computational Intelligence and Neuroscience, 2022. https://doi.org/10.1155/2022/9002713 DOI: https://doi.org/10.1155/2022/9002713
Zitouni, H., Bouchelik, K., Saidi, R., & Chekkai, N. (2020). Personalized Menu: a New Contextual Collaborative Recommender System. 2020 International Conference on Advanced Aspects of Software Engineering (ICAASE), 1-6. https://doi.org/10.1109/ICAASE51408.2020.9380111 DOI: https://doi.org/10.1109/ICAASE51408.2020.9380111
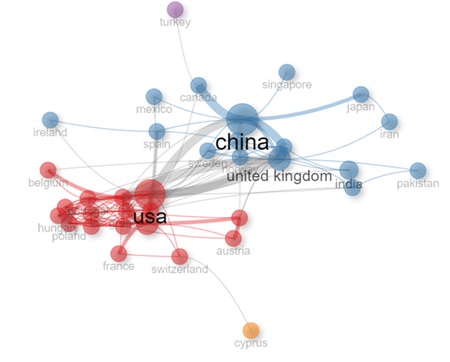
Descargas
Publicado
Cómo citar
Número
Sección
Licencia
Derechos de autor 2023 Erika Del Milagro Lozano-Flores

Esta obra está bajo una licencia internacional Creative Commons Atribución 4.0.
Los autores retienen sus derechos:
a. Los autores retienen sus derechos de marca y patente, y tambien sobre cualquier proceso o procedimiento descrito en el artículo.
b. Los autores retienen el derecho de compartir, copiar, distribuir, ejecutar y comunicar públicamente el articulo publicado en la Revista Científica de Sistemas e Informática (RCSI) (por ejemplo, colocarlo en un repositorio institucional o publicarlo en un libro), con un reconocimiento de su publicación inicial en la RCSI.
c. Los autores retienen el derecho a hacer una posterior publicación de su trabajo, de utilizar el artículo o cualquier parte de aquel (por ejemplo: una compilación de sus trabajos, notas para conferencias, tesis, o para un libro), siempre que indiquen la fuente de publicación (autores del trabajo, revista, volumen, número y fecha).