Advances in the use of artificial intelligence to improve control and fraud detection in organizations
DOI:
https://doi.org/10.51252/rcsi.v3i1.494Keywords:
audit, automated systems, cybersecurity, data analysis, machine learningAbstract
The study reviewed the use of artificial intelligence (AI) to improve fraud control and detection in organizations, based on 31 scientific articles published between 2020 and 2022. Key technologies include machine learning, deep learning, and blockchain, which have been shown to improve the accuracy of fraud detection and optimize the handling of large volumes of data. These tools not only improve internal controls, but also reinforce the security and transparency of transactions, mainly in the financial and business sectors. The results suggest that these technologies reduce false positives and improve real-time detection. However, challenges are identified, such as interoperability between systems and staff training. In conclusion, the adoption of AI in fraud detection is a growing trend that offers advanced solutions, although challenges remain to maximize its long-term impact.
References
Al-Hashedi, K. G., & Magalingam, P. (2021). Financial fraud detection applying data mining techniques: A comprehensive review from 2009 to 2019. Computer Science Review, 40, 100402. https://doi.org/10.1016/j.cosrev.2021.100402 DOI: https://doi.org/10.1016/j.cosrev.2021.100402
Ali, A., Abd Razak, S., Othman, S. H., Eisa, T. A. E., Al-Dhaqm, A., Nasser, M., Elhassan, T., Elshafie, H., & Saif, A. (2022). Financial Fraud Detection Based on Machine Learning: A Systematic Literature Review. Applied Sciences, 12(19), 9637. https://doi.org/10.3390/app12199637 DOI: https://doi.org/10.3390/app12199637
Alotibi, J., Almutanni, B., Alsubait, T., Alhakami, H., & Baz, A. (2022). Money Laundering Detection using Machine Learning and Deep Learning. International Journal of Advanced Computer Science and Applications, 13(10), 732–738. https://doi.org/10.14569/IJACSA.2022.0131087 DOI: https://doi.org/10.14569/IJACSA.2022.0131087
Ashfaq, T., Khalid, R., Yahaya, A. S., Aslam, S., Azar, A. T., Alsafari, S., & Hameed, I. A. (2022). A Machine Learning and Blockchain Based Efficient Fraud Detection Mechanism. Sensors, 22(19). https://doi.org/10.3390/s22197162 DOI: https://doi.org/10.3390/s22197162
Bakumenko, A., & Elragal, A. (2022). Detecting Anomalies in Financial Data Using Machine Learning Algorithms. Systems, 10(5). https://doi.org/10.3390/systems10050130 DOI: https://doi.org/10.3390/systems10050130
Carta, S., Podda, A. S., Recupero, D. R., & Saia, R. (2020). A local feature engineering strategy to improve network anomaly detection. Future Internet, 12(10), 1–30. https://doi.org/10.3390/fi12100177 DOI: https://doi.org/10.3390/fi12100177
Chenoori, R. K., & Kavuri, R. (2022). Online Transaction Fraud Detection Using Efficient Dimensionality Reduction and Machine Learning Techniques. Revue d’Intelligence Artificielle, 36(4), 621–628. https://doi.org/10.18280/ria.360415 DOI: https://doi.org/10.18280/ria.360415
Collins, C., Dennehy, D., Conboy, K., & Mikalef, P. (2021). Artificial intelligence in information systems research: A systematic literature review and research agenda. International Journal of Information Management, 60, 102383. https://doi.org/10.1016/j.ijinfomgt.2021.102383 DOI: https://doi.org/10.1016/j.ijinfomgt.2021.102383
Cremer, F., Sheehan, B., Fortmann, M., Kia, A. N., Mullins, M., Murphy, F., & Materne, S. (2022). Cyber risk and cybersecurity: a systematic review of data availability. The Geneva Papers on Risk and Insurance - Issues and Practice, 47(3), 698–736. https://doi.org/10.1057/s41288-022-00266-6 DOI: https://doi.org/10.1057/s41288-022-00266-6
Cronin, P., Ryan, F., & Coughlan, M. (2008). Undertaking a literature review: a step-by-step approach. British Journal of Nursing, 17(1), 38–43. https://doi.org/10.12968/bjon.2008.17.1.28059 DOI: https://doi.org/10.12968/bjon.2008.17.1.28059
Elsayed, M. A., & Zulkernine, M. (2020). PredictDeep: Security Analytics as a Service for Anomaly Detection and Prediction. IEEE Access, 8, 45184–45197. https://doi.org/10.1109/ACCESS.2020.2977325 DOI: https://doi.org/10.1109/ACCESS.2020.2977325
Engel, C., Ebel, P., & van Giffen, B. (2021). Empirically Exploring the Cause-Effect Relationships of AI Characteristics, Project Management Challenges, and Organizational Change (pp. 166–181). https://doi.org/10.1007/978-3-030-86797-3_12 DOI: https://doi.org/10.1007/978-3-030-86797-3_12
Füller, J., Hutter, K., Wahl, J., Bilgram, V., & Tekic, Z. (2022). How AI revolutionizes innovation management – Perceptions and implementation preferences of AI-based innovators. Technological Forecasting and Social Change, 178, 121598. https://doi.org/10.1016/j.techfore.2022.121598 DOI: https://doi.org/10.1016/j.techfore.2022.121598
Gao, F., Li, J., Cheng, R., Zhou, Y., & Ye, Y. (2021). ConNet: Deep Semi-Supervised Anomaly Detection Based on Sparse Positive Samples. IEEE Access, 9, 67249–67258. https://doi.org/10.1109/ACCESS.2021.3077014 DOI: https://doi.org/10.1109/ACCESS.2021.3077014
Hasnain, M., Jeong, S. R., Pasha, M. F., & Ghani, I. (2020). Performance anomaly detection in web services: An RNN-based approach using dynamic quality of service features. Computers, Materials and Continua, 64(2), 729–752. https://doi.org/10.32604/CMC.2020.010394 DOI: https://doi.org/10.32604/cmc.2020.010394
Hong, S., Wu, H., Xu, X., & Xiong, W. (2022). Early Warning of Enterprise Financial Risk Based on Decision Tree Algorithm. Computational Intelligence and Neuroscience, 2022. https://doi.org/10.1155/2022/9182099 DOI: https://doi.org/10.1155/2022/9182099
Jan, C.-L. (2021). Using deep learning algorithms for CPAs’ going concern prediction. Information (Switzerland), 12(2), 1–22. https://doi.org/10.3390/info12020073 DOI: https://doi.org/10.3390/info12020073
Li, J. (2022). E-Commerce Fraud Detection Model by Computer Artificial Intelligence Data Mining. Computational Intelligence and Neuroscience, 2022. https://doi.org/10.1155/2022/8783783 DOI: https://doi.org/10.1155/2022/8783783
Li, S. L. (2020). Data mining of corporate financial fraud based on neural network model. Computer Optics, 44(4), 665–670. https://doi.org/10.18287/2412-6179-CO-656 DOI: https://doi.org/10.18287/2412-6179-CO-656
Liu, J., Gu, X., & Shang, C. (2020). Quantitative Detection of Financial Fraud Based on Deep Learning with Combination of E-Commerce Big Data. Complexity, 2020. https://doi.org/10.1155/2020/6685888 DOI: https://doi.org/10.1155/2020/6685888
Lois, P., Drogalas, G., Karagiorgos, A., & Tsikalakis, K. (2020). Internal audits in the digital era: opportunities risks and challenges. EuroMed Journal of Business, 15(2), 205–217. https://doi.org/10.1108/EMJB-07-2019-0097 DOI: https://doi.org/10.1108/EMJB-07-2019-0097
Lokanan, M. (2022). The determinants of investment fraud: A machine learning and artificial intelligence approach. Frontiers in Big Data, 5. https://doi.org/10.3389/fdata.2022.961039 DOI: https://doi.org/10.3389/fdata.2022.961039
Maçãs, C., Polisciuc, E., & Machado, P. (2022). ATOVis – A visualisation tool for the detection of financial fraud. Information Visualization, 21(4), 371–392. https://doi.org/10.1177/14738716221098074 DOI: https://doi.org/10.1177/14738716221098074
Mahbub, S., Pardede, E., & Kayes, A. S. M. (2022). Online Recruitment Fraud Detection: A Study on Contextual Features in Australian Job Industries. IEEE Access, 10, 82776–82787. https://doi.org/10.1109/ACCESS.2022.3197225 DOI: https://doi.org/10.1109/ACCESS.2022.3197225
Mani, V., Prakash, M., & Lai, W. C. (2022). Cloud-based blockchain technology to identify counterfeits. Journal of Cloud Computing, 11(1). https://doi.org/10.1186/s13677-022-00341-2 DOI: https://doi.org/10.1186/s13677-022-00341-2
Murorunkwere, B. F., Tuyishimire, O., Haughton, D., & Nzabanita, J. (2022). Fraud Detection Using Neural Networks: A Case Study of Income Tax. Future Internet, 14(6). https://doi.org/10.3390/fi14060168 DOI: https://doi.org/10.3390/fi14060168
Nesvijevskaia, A., Ouillade, S., Guilmin, P., & Zucker, J.-D. (2021). The accuracy versus interpretability trade-off in fraud detection model. Data and Policy, 3(7). https://doi.org/10.1017/dap.2021.3 DOI: https://doi.org/10.1017/dap.2021.3
Ng, K. K. H., Chen, C.-H., Lee, C. K. M., Jiao, J. (Roger), & Yang, Z.-X. (2021). A systematic literature review on intelligent automation: Aligning concepts from theory, practice, and future perspectives. Advanced Engineering Informatics, 47, 101246. https://doi.org/10.1016/j.aei.2021.101246 DOI: https://doi.org/10.1016/j.aei.2021.101246
Omair, B., & Alturki, A. (2020). Multi-dimensional fraud detection metrics in business processes and their application. International Journal of Advanced Computer Science and Applications, 11(9), 570–586. https://doi.org/10.14569/IJACSA.2020.0110968 DOI: https://doi.org/10.14569/IJACSA.2020.0110968
Petrariu, I., Moscaliuc, A., Turcu, C. E., & Gherman, O. (2022). A Comparative Study of Unsupervised Anomaly Detection Algorithms used in a Small and Medium-Sized Enterprise. International Journal of Advanced Computer Science and Applications, 13(9), 931–940. https://doi.org/10.14569/IJACSA.2022.01309108 DOI: https://doi.org/10.14569/IJACSA.2022.01309108
Ponce, E. K., Sanchez, K. E., & Andrade-Arenas, L. (2022). Implementation of a Web System: Prevent Fraud Cases in Electronic Transactions. International Journal of Advanced Computer Science and Applications, 13(6), 865–876. https://doi.org/10.14569/IJACSA.2022.01306102 DOI: https://doi.org/10.14569/IJACSA.2022.01306102
Pranto, T. H., Hasib, K. T. A. M., Rahman, T., Haque, A. B., Islam, A. K. M. N., & Rahman, R. M. (2022). Blockchain and Machine Learning for Fraud Detection: A Privacy-Preserving and Adaptive Incentive Based Approach. IEEE Access, 10, 87115–87134. https://doi.org/10.1109/ACCESS.2022.3198956 DOI: https://doi.org/10.1109/ACCESS.2022.3198956
RB, A., & KR, S. K. (2021). Credit card fraud detection using artificial neural network. Global Transitions Proceedings, 2(1), 35–41. https://doi.org/10.1016/j.gltp.2021.01.006 DOI: https://doi.org/10.1016/j.gltp.2021.01.006
Reim, W., Åström, J., & Eriksson, O. (2020). Implementation of Artificial Intelligence (AI): A Roadmap for Business Model Innovation. AI, 1(2), 180–191. https://doi.org/10.3390/ai1020011 DOI: https://doi.org/10.3390/ai1020011
Rubaidi, Z. S., Ammar, B. B., & Aouicha, M. B. (2022). Fraud Detection Using Large-scale Imbalance Dataset. International Journal on Artificial Intelligence Tools, 31(8). https://doi.org/10.1142/S0218213022500373 DOI: https://doi.org/10.1142/S0218213022500373
Sarno, R., Sinaga, F., & Sungkono, K. R. (2020). Anomaly detection in business processes using process mining and fuzzy association rule learning. Journal of Big Data, 7(1). https://doi.org/10.1186/s40537-019-0277-1 DOI: https://doi.org/10.1186/s40537-019-0277-1
Shi, W., Xu, L., & Peng, D. (2021). Application of Deep Learning in Financial Management Evaluation. Scientific Programming, 2021. https://doi.org/10.1155/2021/2475885 DOI: https://doi.org/10.1155/2021/2475885
Shneiderman, B. (2020). Bridging the Gap Between Ethics and Practice. ACM Transactions on Interactive Intelligent Systems, 10(4), 1–31. https://doi.org/10.1145/3419764 DOI: https://doi.org/10.1145/3419764
Singh, S., Karimipour, H., HaddadPajouh, H., & Dehghantanha, A. (2020). Artificial Intelligence and Security of Industrial Control Systems. In Handbook of Big Data Privacy (pp. 121–164). Springer International Publishing. https://doi.org/10.1007/978-3-030-38557-6_7 DOI: https://doi.org/10.1007/978-3-030-38557-6_7
Stojanović, B., & Božić, J. (2022). Robust Financial Fraud Alerting System Based in the Cloud Environment. Sensors, 22(23). https://doi.org/10.3390/s22239461 DOI: https://doi.org/10.3390/s22239461
Stojanović, B., Božić, J., Hofer-Schmitz, K., Nahrgang, K., Weber, A., Badii, A., Sundaram, M., Jordan, E., & Runevic, J. (2021). Follow the trail: Machine learning for fraud detection in fintech applications. Sensors, 21(5), 1–43. https://doi.org/10.3390/s21051594 DOI: https://doi.org/10.3390/s21051594
Taherdoost, H. (2021). A Review on Risk Management in Information Systems: Risk Policy, Control and Fraud Detection. Electronics, 10(24), 3065. https://doi.org/10.3390/electronics10243065 DOI: https://doi.org/10.3390/electronics10243065
Truong, Y., & Papagiannidis, S. (2022). Artificial intelligence as an enabler for innovation: A review and future research agenda. Technological Forecasting and Social Change, 183, 121852. https://doi.org/10.1016/j.techfore.2022.121852 DOI: https://doi.org/10.1016/j.techfore.2022.121852
Wang, H., Wang, W., Liu, Y., & Alidaee, B. (2022). Integrating Machine Learning Algorithms With Quantum Annealing Solvers for Online Fraud Detection. IEEE Access, 10, 75908–75917. https://doi.org/10.1109/ACCESS.2022.3190897 DOI: https://doi.org/10.1109/ACCESS.2022.3190897
Xu, Y., Liu, X., Cao, X., Huang, C., Liu, E., Qian, S., Liu, X., Wu, Y., Dong, F., Qiu, C.-W., Qiu, J., Hua, K., Su, W., Wu, J., Xu, H., Han, Y., Fu, C., Yin, Z., Liu, M., … Zhang, J. (2021). Artificial intelligence: A powerful paradigm for scientific research. The Innovation, 2(4), 100179. https://doi.org/10.1016/j.xinn.2021.100179 DOI: https://doi.org/10.1016/j.xinn.2021.100179
Zhang, R., & Zheng, L. (2022). Monitoring and Analysis of Venture Capital and Corporate Fraud Based on Deep Learning. Computational Intelligence and Neuroscience, 2022. https://doi.org/10.1155/2022/4589593 DOI: https://doi.org/10.1155/2022/4589593
Zhang, Z., Ma, Y., & Hua, Y. (2022). Financial Fraud Identification Based on Stacking Ensemble Learning Algorithm: Introducing MD&A Text Information. Computational Intelligence and Neuroscience, 2022. https://doi.org/10.1155/2022/1780834 DOI: https://doi.org/10.1155/2022/1780834
Zuiderwijk, A., Chen, Y.-C., & Salem, F. (2021). Implications of the use of artificial intelligence in public governance: A systematic literature review and a research agenda. Government Information Quarterly, 38(3), 101577. https://doi.org/10.1016/j.giq.2021.101577 DOI: https://doi.org/10.1016/j.giq.2021.101577
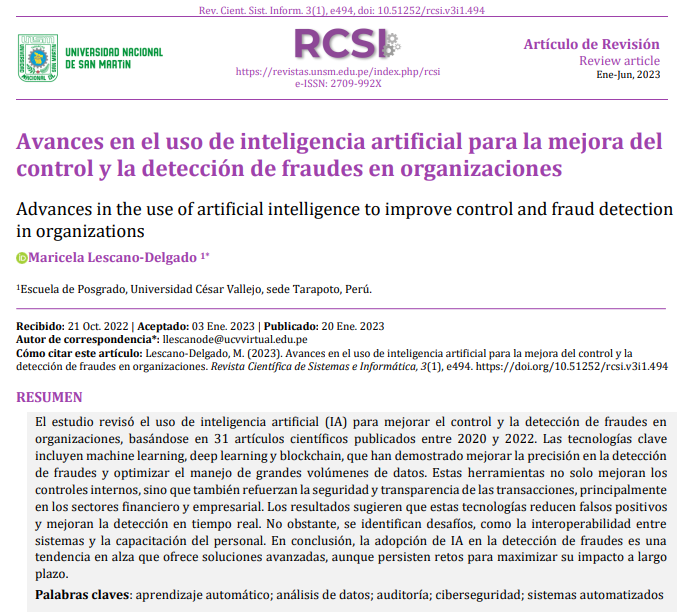
Published
How to Cite
Issue
Section
License
Copyright (c) 2023 Maricela Lescano-Delgado

This work is licensed under a Creative Commons Attribution 4.0 International License.
The authors retain their rights:
a. The authors retain their trademark and patent rights, as well as any process or procedure described in the article.
b. The authors retain the right to share, copy, distribute, execute and publicly communicate the article published in the Revista Científica de Sistemas e Informática (RCSI) (for example, place it in an institutional repository or publish it in a book), with an acknowledgment of its initial publication in the RCSI.
c. Authors retain the right to make a subsequent publication of their work, to use the article or any part of it (for example: a compilation of their works, notes for conferences, thesis, or for a book), provided that they indicate the source of publication (authors of the work, journal, volume, number and date).