Avances en el uso de inteligencia artificial para la mejora del control y la detección de fraudes en organizaciones
DOI:
https://doi.org/10.51252/rcsi.v3i1.494Palabras clave:
aprendizaje automático, análisis de datos, auditoría, ciberseguridad, sistemas automatizadosResumen
El estudio revisó el uso de inteligencia artificial (IA) para mejorar el control y la detección de fraudes en organizaciones, basándose en 31 artículos científicos publicados entre 2020 y 2022. Las tecnologías clave incluyen machine learning, deep learning y blockchain, que han demostrado mejorar la precisión en la detección de fraudes y optimizar el manejo de grandes volúmenes de datos. Estas herramientas no solo mejoran los controles internos, sino que también refuerzan la seguridad y transparencia de las transacciones, principalmente en los sectores financiero y empresarial. Los resultados sugieren que estas tecnologías reducen falsos positivos y mejoran la detección en tiempo real. No obstante, se identifican desafíos, como la interoperabilidad entre sistemas y la capacitación del personal. En conclusión, la adopción de IA en la detección de fraudes es una tendencia en alza que ofrece soluciones avanzadas, aunque persisten retos para maximizar su impacto a largo plazo.
Citas
Al-Hashedi, K. G., & Magalingam, P. (2021). Financial fraud detection applying data mining techniques: A comprehensive review from 2009 to 2019. Computer Science Review, 40, 100402. https://doi.org/10.1016/j.cosrev.2021.100402 DOI: https://doi.org/10.1016/j.cosrev.2021.100402
Ali, A., Abd Razak, S., Othman, S. H., Eisa, T. A. E., Al-Dhaqm, A., Nasser, M., Elhassan, T., Elshafie, H., & Saif, A. (2022). Financial Fraud Detection Based on Machine Learning: A Systematic Literature Review. Applied Sciences, 12(19), 9637. https://doi.org/10.3390/app12199637 DOI: https://doi.org/10.3390/app12199637
Alotibi, J., Almutanni, B., Alsubait, T., Alhakami, H., & Baz, A. (2022). Money Laundering Detection using Machine Learning and Deep Learning. International Journal of Advanced Computer Science and Applications, 13(10), 732–738. https://doi.org/10.14569/IJACSA.2022.0131087 DOI: https://doi.org/10.14569/IJACSA.2022.0131087
Ashfaq, T., Khalid, R., Yahaya, A. S., Aslam, S., Azar, A. T., Alsafari, S., & Hameed, I. A. (2022). A Machine Learning and Blockchain Based Efficient Fraud Detection Mechanism. Sensors, 22(19). https://doi.org/10.3390/s22197162 DOI: https://doi.org/10.3390/s22197162
Bakumenko, A., & Elragal, A. (2022). Detecting Anomalies in Financial Data Using Machine Learning Algorithms. Systems, 10(5). https://doi.org/10.3390/systems10050130 DOI: https://doi.org/10.3390/systems10050130
Carta, S., Podda, A. S., Recupero, D. R., & Saia, R. (2020). A local feature engineering strategy to improve network anomaly detection. Future Internet, 12(10), 1–30. https://doi.org/10.3390/fi12100177 DOI: https://doi.org/10.3390/fi12100177
Chenoori, R. K., & Kavuri, R. (2022). Online Transaction Fraud Detection Using Efficient Dimensionality Reduction and Machine Learning Techniques. Revue d’Intelligence Artificielle, 36(4), 621–628. https://doi.org/10.18280/ria.360415 DOI: https://doi.org/10.18280/ria.360415
Collins, C., Dennehy, D., Conboy, K., & Mikalef, P. (2021). Artificial intelligence in information systems research: A systematic literature review and research agenda. International Journal of Information Management, 60, 102383. https://doi.org/10.1016/j.ijinfomgt.2021.102383 DOI: https://doi.org/10.1016/j.ijinfomgt.2021.102383
Cremer, F., Sheehan, B., Fortmann, M., Kia, A. N., Mullins, M., Murphy, F., & Materne, S. (2022). Cyber risk and cybersecurity: a systematic review of data availability. The Geneva Papers on Risk and Insurance - Issues and Practice, 47(3), 698–736. https://doi.org/10.1057/s41288-022-00266-6 DOI: https://doi.org/10.1057/s41288-022-00266-6
Cronin, P., Ryan, F., & Coughlan, M. (2008). Undertaking a literature review: a step-by-step approach. British Journal of Nursing, 17(1), 38–43. https://doi.org/10.12968/bjon.2008.17.1.28059 DOI: https://doi.org/10.12968/bjon.2008.17.1.28059
Elsayed, M. A., & Zulkernine, M. (2020). PredictDeep: Security Analytics as a Service for Anomaly Detection and Prediction. IEEE Access, 8, 45184–45197. https://doi.org/10.1109/ACCESS.2020.2977325 DOI: https://doi.org/10.1109/ACCESS.2020.2977325
Engel, C., Ebel, P., & van Giffen, B. (2021). Empirically Exploring the Cause-Effect Relationships of AI Characteristics, Project Management Challenges, and Organizational Change (pp. 166–181). https://doi.org/10.1007/978-3-030-86797-3_12 DOI: https://doi.org/10.1007/978-3-030-86797-3_12
Füller, J., Hutter, K., Wahl, J., Bilgram, V., & Tekic, Z. (2022). How AI revolutionizes innovation management – Perceptions and implementation preferences of AI-based innovators. Technological Forecasting and Social Change, 178, 121598. https://doi.org/10.1016/j.techfore.2022.121598 DOI: https://doi.org/10.1016/j.techfore.2022.121598
Gao, F., Li, J., Cheng, R., Zhou, Y., & Ye, Y. (2021). ConNet: Deep Semi-Supervised Anomaly Detection Based on Sparse Positive Samples. IEEE Access, 9, 67249–67258. https://doi.org/10.1109/ACCESS.2021.3077014 DOI: https://doi.org/10.1109/ACCESS.2021.3077014
Hasnain, M., Jeong, S. R., Pasha, M. F., & Ghani, I. (2020). Performance anomaly detection in web services: An RNN-based approach using dynamic quality of service features. Computers, Materials and Continua, 64(2), 729–752. https://doi.org/10.32604/CMC.2020.010394 DOI: https://doi.org/10.32604/cmc.2020.010394
Hong, S., Wu, H., Xu, X., & Xiong, W. (2022). Early Warning of Enterprise Financial Risk Based on Decision Tree Algorithm. Computational Intelligence and Neuroscience, 2022. https://doi.org/10.1155/2022/9182099 DOI: https://doi.org/10.1155/2022/9182099
Jan, C.-L. (2021). Using deep learning algorithms for CPAs’ going concern prediction. Information (Switzerland), 12(2), 1–22. https://doi.org/10.3390/info12020073 DOI: https://doi.org/10.3390/info12020073
Li, J. (2022). E-Commerce Fraud Detection Model by Computer Artificial Intelligence Data Mining. Computational Intelligence and Neuroscience, 2022. https://doi.org/10.1155/2022/8783783 DOI: https://doi.org/10.1155/2022/8783783
Li, S. L. (2020). Data mining of corporate financial fraud based on neural network model. Computer Optics, 44(4), 665–670. https://doi.org/10.18287/2412-6179-CO-656 DOI: https://doi.org/10.18287/2412-6179-CO-656
Liu, J., Gu, X., & Shang, C. (2020). Quantitative Detection of Financial Fraud Based on Deep Learning with Combination of E-Commerce Big Data. Complexity, 2020. https://doi.org/10.1155/2020/6685888 DOI: https://doi.org/10.1155/2020/6685888
Lois, P., Drogalas, G., Karagiorgos, A., & Tsikalakis, K. (2020). Internal audits in the digital era: opportunities risks and challenges. EuroMed Journal of Business, 15(2), 205–217. https://doi.org/10.1108/EMJB-07-2019-0097 DOI: https://doi.org/10.1108/EMJB-07-2019-0097
Lokanan, M. (2022). The determinants of investment fraud: A machine learning and artificial intelligence approach. Frontiers in Big Data, 5. https://doi.org/10.3389/fdata.2022.961039 DOI: https://doi.org/10.3389/fdata.2022.961039
Maçãs, C., Polisciuc, E., & Machado, P. (2022). ATOVis – A visualisation tool for the detection of financial fraud. Information Visualization, 21(4), 371–392. https://doi.org/10.1177/14738716221098074 DOI: https://doi.org/10.1177/14738716221098074
Mahbub, S., Pardede, E., & Kayes, A. S. M. (2022). Online Recruitment Fraud Detection: A Study on Contextual Features in Australian Job Industries. IEEE Access, 10, 82776–82787. https://doi.org/10.1109/ACCESS.2022.3197225 DOI: https://doi.org/10.1109/ACCESS.2022.3197225
Mani, V., Prakash, M., & Lai, W. C. (2022). Cloud-based blockchain technology to identify counterfeits. Journal of Cloud Computing, 11(1). https://doi.org/10.1186/s13677-022-00341-2 DOI: https://doi.org/10.1186/s13677-022-00341-2
Murorunkwere, B. F., Tuyishimire, O., Haughton, D., & Nzabanita, J. (2022). Fraud Detection Using Neural Networks: A Case Study of Income Tax. Future Internet, 14(6). https://doi.org/10.3390/fi14060168 DOI: https://doi.org/10.3390/fi14060168
Nesvijevskaia, A., Ouillade, S., Guilmin, P., & Zucker, J.-D. (2021). The accuracy versus interpretability trade-off in fraud detection model. Data and Policy, 3(7). https://doi.org/10.1017/dap.2021.3 DOI: https://doi.org/10.1017/dap.2021.3
Ng, K. K. H., Chen, C.-H., Lee, C. K. M., Jiao, J. (Roger), & Yang, Z.-X. (2021). A systematic literature review on intelligent automation: Aligning concepts from theory, practice, and future perspectives. Advanced Engineering Informatics, 47, 101246. https://doi.org/10.1016/j.aei.2021.101246 DOI: https://doi.org/10.1016/j.aei.2021.101246
Omair, B., & Alturki, A. (2020). Multi-dimensional fraud detection metrics in business processes and their application. International Journal of Advanced Computer Science and Applications, 11(9), 570–586. https://doi.org/10.14569/IJACSA.2020.0110968 DOI: https://doi.org/10.14569/IJACSA.2020.0110968
Petrariu, I., Moscaliuc, A., Turcu, C. E., & Gherman, O. (2022). A Comparative Study of Unsupervised Anomaly Detection Algorithms used in a Small and Medium-Sized Enterprise. International Journal of Advanced Computer Science and Applications, 13(9), 931–940. https://doi.org/10.14569/IJACSA.2022.01309108 DOI: https://doi.org/10.14569/IJACSA.2022.01309108
Ponce, E. K., Sanchez, K. E., & Andrade-Arenas, L. (2022). Implementation of a Web System: Prevent Fraud Cases in Electronic Transactions. International Journal of Advanced Computer Science and Applications, 13(6), 865–876. https://doi.org/10.14569/IJACSA.2022.01306102 DOI: https://doi.org/10.14569/IJACSA.2022.01306102
Pranto, T. H., Hasib, K. T. A. M., Rahman, T., Haque, A. B., Islam, A. K. M. N., & Rahman, R. M. (2022). Blockchain and Machine Learning for Fraud Detection: A Privacy-Preserving and Adaptive Incentive Based Approach. IEEE Access, 10, 87115–87134. https://doi.org/10.1109/ACCESS.2022.3198956 DOI: https://doi.org/10.1109/ACCESS.2022.3198956
RB, A., & KR, S. K. (2021). Credit card fraud detection using artificial neural network. Global Transitions Proceedings, 2(1), 35–41. https://doi.org/10.1016/j.gltp.2021.01.006 DOI: https://doi.org/10.1016/j.gltp.2021.01.006
Reim, W., Åström, J., & Eriksson, O. (2020). Implementation of Artificial Intelligence (AI): A Roadmap for Business Model Innovation. AI, 1(2), 180–191. https://doi.org/10.3390/ai1020011 DOI: https://doi.org/10.3390/ai1020011
Rubaidi, Z. S., Ammar, B. B., & Aouicha, M. B. (2022). Fraud Detection Using Large-scale Imbalance Dataset. International Journal on Artificial Intelligence Tools, 31(8). https://doi.org/10.1142/S0218213022500373 DOI: https://doi.org/10.1142/S0218213022500373
Sarno, R., Sinaga, F., & Sungkono, K. R. (2020). Anomaly detection in business processes using process mining and fuzzy association rule learning. Journal of Big Data, 7(1). https://doi.org/10.1186/s40537-019-0277-1 DOI: https://doi.org/10.1186/s40537-019-0277-1
Shi, W., Xu, L., & Peng, D. (2021). Application of Deep Learning in Financial Management Evaluation. Scientific Programming, 2021. https://doi.org/10.1155/2021/2475885 DOI: https://doi.org/10.1155/2021/2475885
Shneiderman, B. (2020). Bridging the Gap Between Ethics and Practice. ACM Transactions on Interactive Intelligent Systems, 10(4), 1–31. https://doi.org/10.1145/3419764 DOI: https://doi.org/10.1145/3419764
Singh, S., Karimipour, H., HaddadPajouh, H., & Dehghantanha, A. (2020). Artificial Intelligence and Security of Industrial Control Systems. In Handbook of Big Data Privacy (pp. 121–164). Springer International Publishing. https://doi.org/10.1007/978-3-030-38557-6_7 DOI: https://doi.org/10.1007/978-3-030-38557-6_7
Stojanović, B., & Božić, J. (2022). Robust Financial Fraud Alerting System Based in the Cloud Environment. Sensors, 22(23). https://doi.org/10.3390/s22239461 DOI: https://doi.org/10.3390/s22239461
Stojanović, B., Božić, J., Hofer-Schmitz, K., Nahrgang, K., Weber, A., Badii, A., Sundaram, M., Jordan, E., & Runevic, J. (2021). Follow the trail: Machine learning for fraud detection in fintech applications. Sensors, 21(5), 1–43. https://doi.org/10.3390/s21051594 DOI: https://doi.org/10.3390/s21051594
Taherdoost, H. (2021). A Review on Risk Management in Information Systems: Risk Policy, Control and Fraud Detection. Electronics, 10(24), 3065. https://doi.org/10.3390/electronics10243065 DOI: https://doi.org/10.3390/electronics10243065
Truong, Y., & Papagiannidis, S. (2022). Artificial intelligence as an enabler for innovation: A review and future research agenda. Technological Forecasting and Social Change, 183, 121852. https://doi.org/10.1016/j.techfore.2022.121852 DOI: https://doi.org/10.1016/j.techfore.2022.121852
Wang, H., Wang, W., Liu, Y., & Alidaee, B. (2022). Integrating Machine Learning Algorithms With Quantum Annealing Solvers for Online Fraud Detection. IEEE Access, 10, 75908–75917. https://doi.org/10.1109/ACCESS.2022.3190897 DOI: https://doi.org/10.1109/ACCESS.2022.3190897
Xu, Y., Liu, X., Cao, X., Huang, C., Liu, E., Qian, S., Liu, X., Wu, Y., Dong, F., Qiu, C.-W., Qiu, J., Hua, K., Su, W., Wu, J., Xu, H., Han, Y., Fu, C., Yin, Z., Liu, M., … Zhang, J. (2021). Artificial intelligence: A powerful paradigm for scientific research. The Innovation, 2(4), 100179. https://doi.org/10.1016/j.xinn.2021.100179 DOI: https://doi.org/10.1016/j.xinn.2021.100179
Zhang, R., & Zheng, L. (2022). Monitoring and Analysis of Venture Capital and Corporate Fraud Based on Deep Learning. Computational Intelligence and Neuroscience, 2022. https://doi.org/10.1155/2022/4589593 DOI: https://doi.org/10.1155/2022/4589593
Zhang, Z., Ma, Y., & Hua, Y. (2022). Financial Fraud Identification Based on Stacking Ensemble Learning Algorithm: Introducing MD&A Text Information. Computational Intelligence and Neuroscience, 2022. https://doi.org/10.1155/2022/1780834 DOI: https://doi.org/10.1155/2022/1780834
Zuiderwijk, A., Chen, Y.-C., & Salem, F. (2021). Implications of the use of artificial intelligence in public governance: A systematic literature review and a research agenda. Government Information Quarterly, 38(3), 101577. https://doi.org/10.1016/j.giq.2021.101577 DOI: https://doi.org/10.1016/j.giq.2021.101577
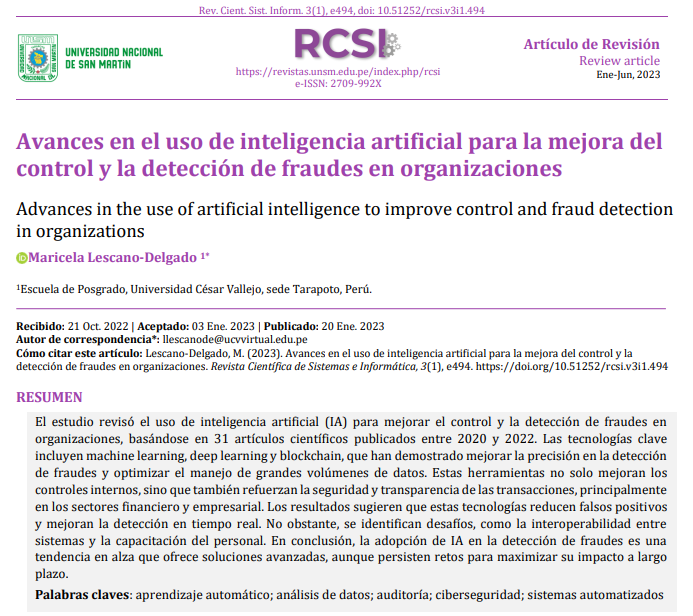
Publicado
Cómo citar
Número
Sección
Licencia
Derechos de autor 2023 Maricela Lescano-Delgado

Esta obra está bajo una licencia internacional Creative Commons Atribución 4.0.
Los autores retienen sus derechos:
a. Los autores retienen sus derechos de marca y patente, y tambien sobre cualquier proceso o procedimiento descrito en el artículo.
b. Los autores retienen el derecho de compartir, copiar, distribuir, ejecutar y comunicar públicamente el articulo publicado en la Revista Científica de Sistemas e Informática (RCSI) (por ejemplo, colocarlo en un repositorio institucional o publicarlo en un libro), con un reconocimiento de su publicación inicial en la RCSI.
c. Los autores retienen el derecho a hacer una posterior publicación de su trabajo, de utilizar el artículo o cualquier parte de aquel (por ejemplo: una compilación de sus trabajos, notas para conferencias, tesis, o para un libro), siempre que indiquen la fuente de publicación (autores del trabajo, revista, volumen, número y fecha).